Generative AI
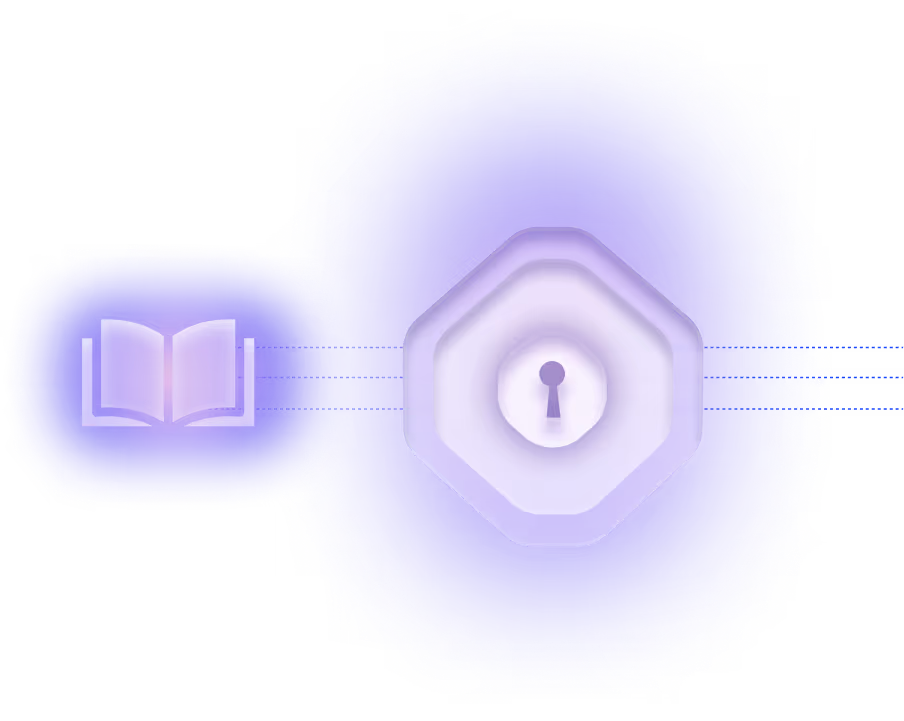
What is Generative AI?
Generative AI refers to a class of artificial intelligence models capable of producing new content, such as text, code, images, or audio, based on patterns learned from large volumes of training data. Powered by architectures like large language models (LLMs) and diffusion networks, Generative AI systems can perform complex tasks including summarization, translation, code generation, and decision support. In cybersecurity and identity management, Generative AI is increasingly embedded into operational workflows for threat detection, incident response, and automation.
Why is it important?
Generative AI has fundamentally reshaped how enterprises approach automation, data analysis, and cybersecurity. In modern cloud-native and hybrid environments, it enables scalable solutions for real-time alert triage, risk scoring, and remediation. However, its effectiveness depends heavily on access to internal data, APIs, and services—requiring the use of Non-Human Identities (NHIs) such as service accounts, API keys, and machine credentials. This dependency introduces a new set of security concerns, where the misuse or compromise of these identities can result in prompt injection, data poisoning, or unauthorized access to sensitive systems.
What are common applications or use cases?
In practice, Generative AI is used to enhance security operations by generating real-time threat intelligence, summarizing incident reports, and automating playbooks. For example, a GenAI-powered tool may use NHIs to query internal data sources and generate contextual threat assessments. In development environments, GenAI assists with secure code generation and vulnerability detection. Enterprises also leverage Retrieval-Augmented Generation (RAG) to provide AI with access to proprietary datasets, enhancing the accuracy and relevance of outputs. However, each of these use cases relies on properly scoped and secured NHIs to prevent abuse.
What is the connection to NHIs (Non-Human Identities)?
Generative AI systems depend on NHIs to access data, invoke cloud services, and integrate with enterprise systems. These NHIs often hold elevated privileges and operate without human oversight, making them high-value targets for attackers. Threats such as model theft, lateral movement, and disinformation campaigns often stem from compromised NHIs used in GenAI pipelines. For instance, a hijacked API key could enable a malicious actor to poison training data or execute unauthorized queries via an AI assistant integrated into internal systems.
Are there any notable industry data, trends, or standards?
Yes. Industry reports indicate that nearly 68% of cloud breaches involve compromised machine credentials—many of which are tied to AI systems. Incidents such as the 2023 Microsoft SAS token exposure and the 2024 Samsung data leaks illustrate the high risk of unmanaged NHIs in GenAI contexts. Regulatory frameworks like the EU AI Act and NIST’s AI Risk Management Framework now emphasize the need for auditable controls over AI access and identity governance. Best practices include least-privilege enforcement, automated credential rotation, and anomaly detection tailored to NHI behavior in AI workflows.
What is the broader impact or takeaway?
Generative AI offers transformative benefits for enterprise security and operations, but its safe deployment depends on securing the NHIs that fuel it. Organizations must adopt a layered defense strategy that includes NHI lifecycle automation, policy-based access controls, and real-time behavioral analytics. As regulatory pressure increases and AI adoption accelerates, aligning GenAI initiatives with robust NHI governance will be critical to maintaining trust, compliance, and operational integrity in modern digital infrastructures.